Migration Games
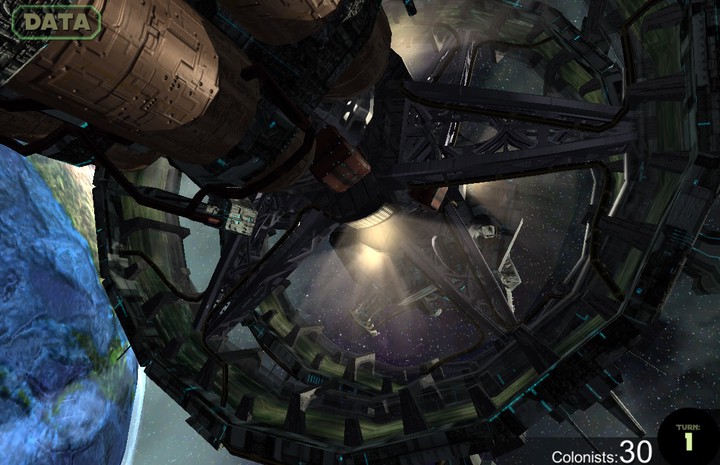
Migration is difficult to study because there is so little data. People tend not to move: an estimated 3% of the world’s population ever migrates. And when people do move, they rarely leave forwarding addresses. Despite the increasing proliferation of large socioeconomic survey datasets, talking to people at their homes doesn’t tell us much about what happened to the ones who migrated, or why they left. We do know that people move for a variety of reasons, usually as a way of seeking a better life—higher wages, more education, easier access to healthcare, more freedom, more opportunity generally. But we know little about how and why people make that exact decision over whether and when to stay or go.
Immobility is puzzling to many economists because it appears that many people might significantly their quality of life by moving. Even small changes in location, moving across zip codes and neighborhoods, can yield large improvements in a person’s safety and economic outcomes. Of course, people have a strong attachment to their homes—so maybe this is what keeps them put? Or is the belief that economists have, that so many people could upgrade their lives by moving, just an illusion? After all, if these moves were really so beneficial, why haven’t people already made them?
A Lab Experiment
To understand how people migrate I needed an experiment where I could condense a lifetime of migration choices down to a more manageable timeframe, and where I could actually know their payoff to moving, not just guess at it from their observed demographics. That way I could answer the question whether people really were forgoing significant economic opportunity by not migrating.
The structure of a migration decision is very simple: you decide whether to stay where you are or to leave, and if you choose to leave, you decide where to go. You make this decision, repeatedly, and you learn over time whether you’ve made the right decision. Staying where you are might be what’s best for you; maybe moving to a new town or state would offer you a better life? Recreating the magnitude of this choice in an experimental setting is difficult because where we choose to live is one of the biggest decisions we ever make in our lives.
Yet there are instances where people become hugely dedicated to solving virtual problems. These are computer and video games, which people play furiously, competitively—and not necessarily with the expectation of any monetary reward. I thought about games I played as a kid, like The Oregon Trail, where I worked hard to guide a family on a historic, cross-continental migration.
I decided to design a similar migration video game. However, I wanted to isolate the stay-or-go and where-to-go decision-making process at the root of migration. I didn’t want to make the game too literal about migration, as I was worried about it falling into the “uncanny valley:” the more the game is explicitly about migration, the more obvious it may be to players that they are not actually migrating. Some abstraction was necessary. I also wanted to unmoor players from their prior beliefs. For example, if I show you a map, even of a fictional land, some places will look better than others depending on your own idiosyncratic preferences.
In order to create a migration game that fell outside of the uncanny valley and abstracted away from individual preferences, I set the game in outer space, a place that no-one in my study would have significant priors over. The game involves players captaining a starship, moving it between alien worlds. The concept is outlandish, but also provides a compelling frame for optimal dynamic problem solving that is disconnected from participant’s own experiences. When people play this game, they are solving a migration problem, they are moving between worlds finding the one they do the best at.
Testing the game
To gather data on decision-making in vastly different contexts I recruited participants in two countries. In the United States I ran the game at the University of Wisconsin-Madison’s BRITE Lab, and in Ethiopia I ran the game at the University of Bahir Dar. Each participant played the game for approximately 30 minutes to an hour, then filled out a detailed demographic survey.
Findings
The migration game presents players with a reinforcement learning problem under uncertainty. Players attempt to maximize their rewards by moving between worlds, discovering over time which one best suits them. The structure of this game allows me to solve it with a multi-armed bandit algorithm.1 These are reinforcement learning algorithms that find optimal solutions to repeated problems over time, even if they don’t have any prior information as to what the problem looks like. This makes their solutions easily interpretable to people, but also a fair comparison to human problem solving, as they face the same information constraints that people do.
I find that while multi-armed bandit algorithms and people solve the game in different ways, their outcomes are almost identical. Given nothing more than an objective, to get the highest score, both people and algorithms reach an optimal solution. To be clear, this high score is not the same one if they started out with full information. But when uncertainty over the payoff to different moves is high, people appear to make the right choice over when to stay or go. This means that much of the immobility we see is not the result of barriers to migration, so much as limited information of what our lives would be like had we made different choices.
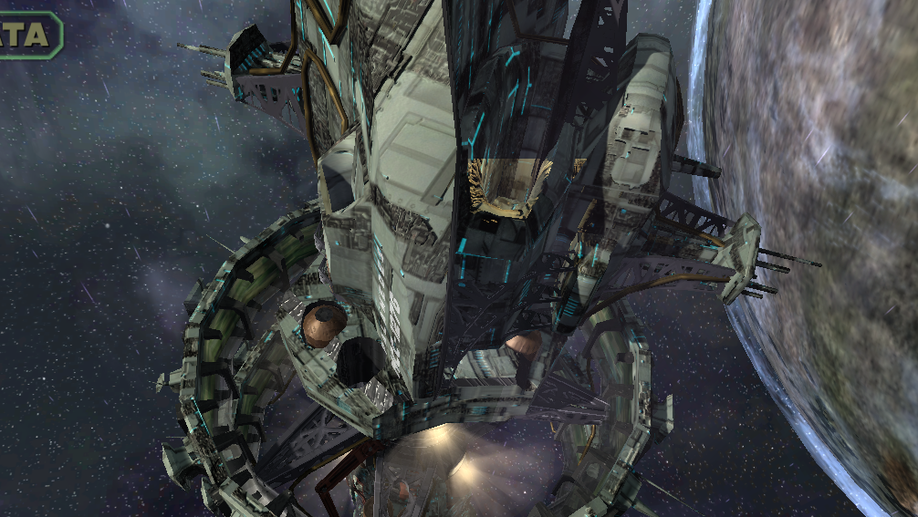
Should I Stay or Should I Go: Microeconomic Determinants of Migration
A video game to study how people make economic migration decisions
-
The term “multi-armed bandit” is a reference to an obscure colloquialism for slot machines as “one armed bandits,” i.e. they had an arm you would pull and they would take your money. An algorithm that could find the right strategy for playing N slot machines would then be a multi-armed bandit. ↩︎